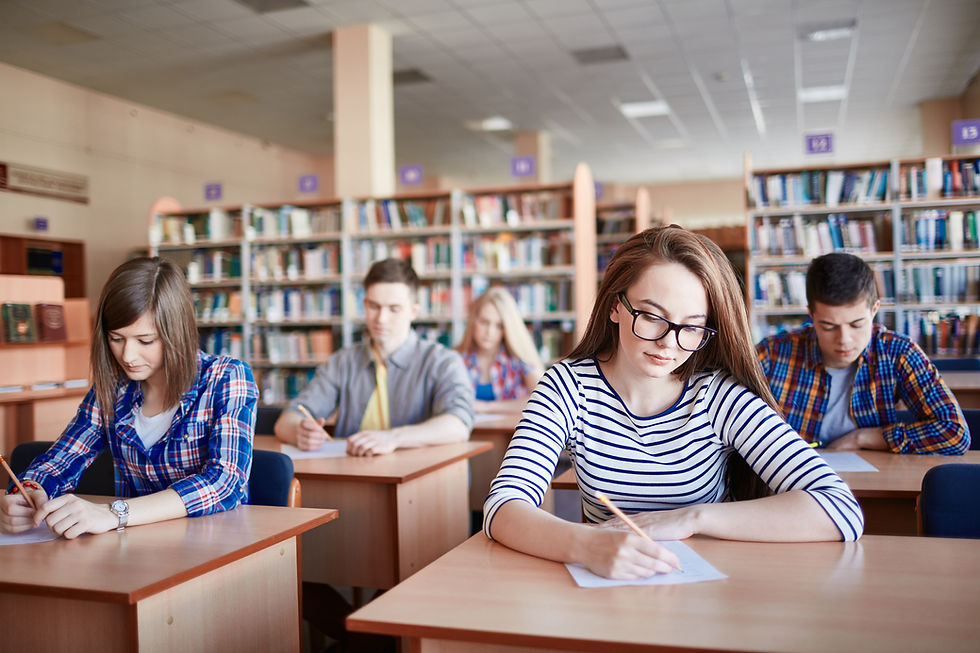
Multi-sensor Data Fusion for
Wearable Respiratory Rate Monitoring Microsystem
Senior Design Project

Problem Statement
Nowadays, respiratory rate is a vital sign used to monitor the progression of illness. An abnormal respiratory rate (RR) is an important marker of serious illness. Various methods have been explored for respiratory rate measurement. These include contact methods based on measurement of chest and abdominal movements, acoustic sounds and airflow, exhaled carbon dioxide and calculation of RR from electrocardiogram (ECG) or oxygen saturation (i.e., photoplethysmography or PPG).
Current Solutions
Abstract
This project aims at improving accuracy of a wearable respiratory rate (RR) monitoring microsystem by data fusion of outputs from RR sensors based on microphone and oximeter. Different algorithms are evaluated and applied for situations with and without reference data. Kalman Filter, relevance coefficient, covariance, Mahalanobis distance and multiple linear regression are applied to realize an optimized algorithm for data fusion.
Team Members
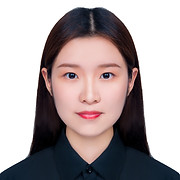
Wenwen He
B. S. Student in EE

Peixuan Jiang
B. S. Student in EE

Hongdan Yang
B. S. Student in EE
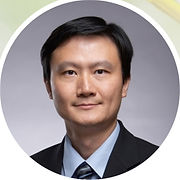
Tao Li, Ph. D.
Advisor
Acknowledgements
Dr. Tao Li’s Autonomous Integrated Microsystems (AIM) Laboratory
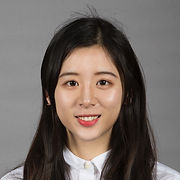_edited.jpg)
Yue Sun
M. S. Student in EE
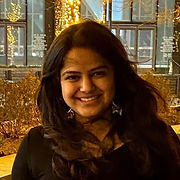_edited.jpg)
Jayasheel Gowda Greeshma
M. S. Student in EE